As our product further develops and I get to see how it is turning out, it seems that a another and different competitive advantage is beginning to emerge. One that I was not expecting as we aimed to find solutions for just small business. Perhaps I am influenced by thoughts of pivoting to the B2E space. Perhaps it is just the journey where you do not always end up where you thought you would.
Observing consultants and IT organizations implement large business intelligence solutions, I find often that the first project either fails entirely or never gets past the initial phases. Lessons learned from these setbacks are often rooted in the client/ business not knowing the data they really want or political battles over which information and metrics are most important. Technical architects have avoided such battles by instead providing data marts, so that managers can help themselves to any data and build metrics in a self-service manner. By giving the client or business everything, the problem is solved.
But does this approach really help the business in the end? My answer is no. Managers remain misaligned- serving their own agendas instead of those of the overall business. Internal to the organization, data proliferation occurs where meanings get blurred and maintenance is so difficult even labels lose their original purpose of a sufficient description. Data silos of big data proportions are saved per division which is wasteful and duplicative.
Generally, I am describing an enterprise problem. Small and medium-sized businesses (SMB) suffer less from these political problems as they cannot afford much system customization and the resources needed to maintain it. Instead the SMBs tend to stick with the standard and best practice fields and data points. Because of this, Bimotics can provide our customers a solution we call the “gallery of analytics”. This gallery hosts all the business analytics available given the operational and financial application data marts for which the customer has data. Generally, these pre-built analytics reflect best practice operational and sales processes that is fundamental in all business looking to grow. The image below is an example of what the analytics gallery looks like. 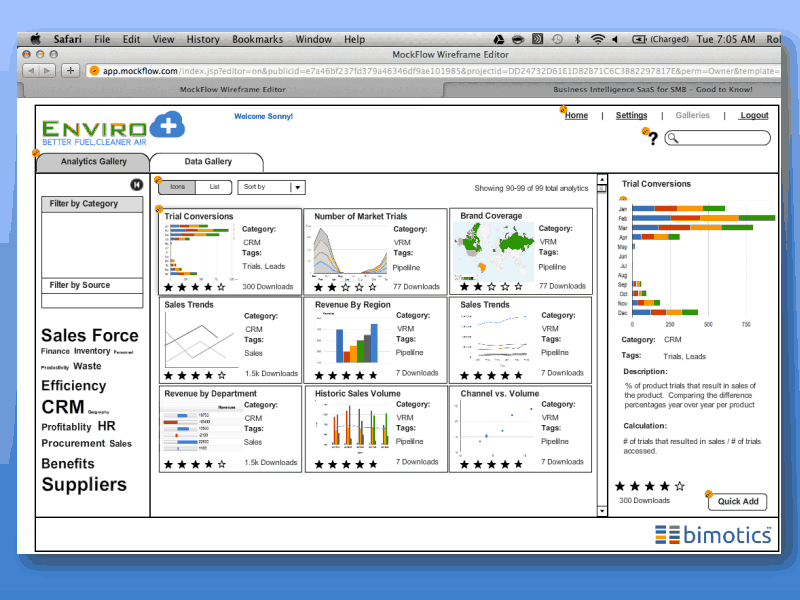
The value to business owners is that they do not need to know what metrics they want before they bring on analytics. Instead they pick and choose the available analytics that which makes sense to answer a particular business problem. They also can prioritize these analytics based on the business strategy they laid out for now. If the business changes direction, then the business owner can change out the analytics to reflect this new vision. Not having to go back to the drawing board saves precious time. This gallery approach to analytics puts the definition and the prioritization of metrics and at the end as well as provides breadth and flexibility to a manager.
Can this same principle be applied to solve an enterprise problem? Although very complex to build, can an “analytics mart” based on only the standard fields and best practice processes of major enterprise applications such as SAP, Oracle, Microsoft and SalesForce be built using the similar principles as our “gallery of analytics”? This “analytics mart” would cut across the different vendors so that advanced metrics are available. For example, metrics, like support center effectiveness, are shown as a blend of financials in SAP with support data from Siebel. This proposed approach, solves amongst the greatest barriers that keep enterprises from successfully implementing business intelligence in that it defines what to measure up front and avoids the interdepartmental politics in its allegiance to only standard and best practice processes.
Why companies have not implemented “analytics marts” already? The answer is twofold. First building an analytic mart across enterprise systems houses many technical complexities especially when looking at all the software versions and system customizations that exist per enterprise. This is not to say that a solution is technically impossible however. Second, budgets split by division and departments need to be continuously spent in full which enable data silo behavior over cross department collaboration and process analysis. In other words, large organizations have budgetary policies that encourages managers buy solutions while wearing horse blinders. I wonder how much of a fundamental shift would need to occur in an organization to embrace data and metrics sharing.
The key to customer adoption of the “analytics mart” solution rests on a consolidated drive to improve the overall business and the willingness of enterprise managers collaborate holistically. Will these managers be courageous enough to be measured against fundamental business process standards in addition to how well their divisions support the corporate strategy at hand?
No comments:
Post a Comment